Rice (Oryza sativa, also African rice, O. glaberrima) is the most important staple food for most of the world and it is especially important in Asia. Unlike sugar cane and maize, the two crops with higher global production, rice is primarily consumed directly as food. Thus, it occupies a uniquely critical position for global food security.
The CERES-Rice module of the Cropping System Model (CSM) of DSSAT was initially developed by an interdisciplinary team of scientists from Michigan State University (MSU), the International Fertilizer Development Center (IFDC), and the University of Hawaii. Joe Ritchie, then at MSU and Upendra Singh at IFDC coordinated the development of the model (Singh et al. 1990; Buresh et al. 1991; Singh et al., 1993; Ritchie, et al., 1987, 1998).
Similar to other crop modules of CSM, the model uses a minimum of readily available daily weather data, soil profile characteristics, crop management, and variety-specific genetic inputs. To simulate rice growth, development, and yield the model considers the following processes:
- Rice development, especially as affected by genotype and weather. The model simulates the effects of photoperiod and temperature on the timing of panicle initiation and the duration of each major growth stage. A provision has been made within the model to calculate an effect of transplanting shock on phase duration.
- Extension growth of leaves, stems, and roots.
- Biomass accumulation using a CERES-type radiation use efficiency approach that includes the effect of atmospheric CO2 concentration.
- Biomass partitioning using phenology to drive the development and growth of vegetative and reproductive organs.
- Stress effects of low temperature causing loss of floret fertility (Godwin et al., 1994).
- Water balance that simulates the daily evaporation, runoff, percolation, and crop water uptake under fully irrigated conditions, rainfed conditions with intermittent flooding and drying, and fully upland conditions where the soil is never flooded. The latter code is shared with all CSM crop modules.(Ritchie, 1998)
- Soil and floodwater nitrogen transformations associated with mineralization/immobilization, urea hydrolysis, nitrification, denitrification, ammonia volatilization, losses of N associated with runoff and percolation, and uptake and utilization of N by the crop, shared with all CSM crop modules (Godwin and Singh, 1998)
As a process-based model, CERES-Rice can be applied to a wide range of research problems, including response to irrigation (Ahmad et al., 2013), nitrogen (Ahmad et al., 2012; Zhang et al., 2018), effects of environmental conditions (Phakamas et al., 2013), rice-wheat production systems (Ahmad et al., 2019; Devkota et al., 2015), yield-gap analyses (Singh et al., 2016; Zhang et al., 2018), yield forecasting (Jha et al., 2019; Kaeomuangmoon et al., 2019), and breeding and rice genetics (Gao et al., 2019; Buddhaboon et al., 2018). This includes potential impacts of climate change (Ahmad et al., 2019; Singh and Ritchie, 1993; Gupta and Mishra, 2019; Nasir et al., 2020). CERES-Rice has participated extensively in AgMIP-related model intercomparisons (e.g., Li et al., 2014; Hasegawa et al., 2017; Wallach et al., 2018).
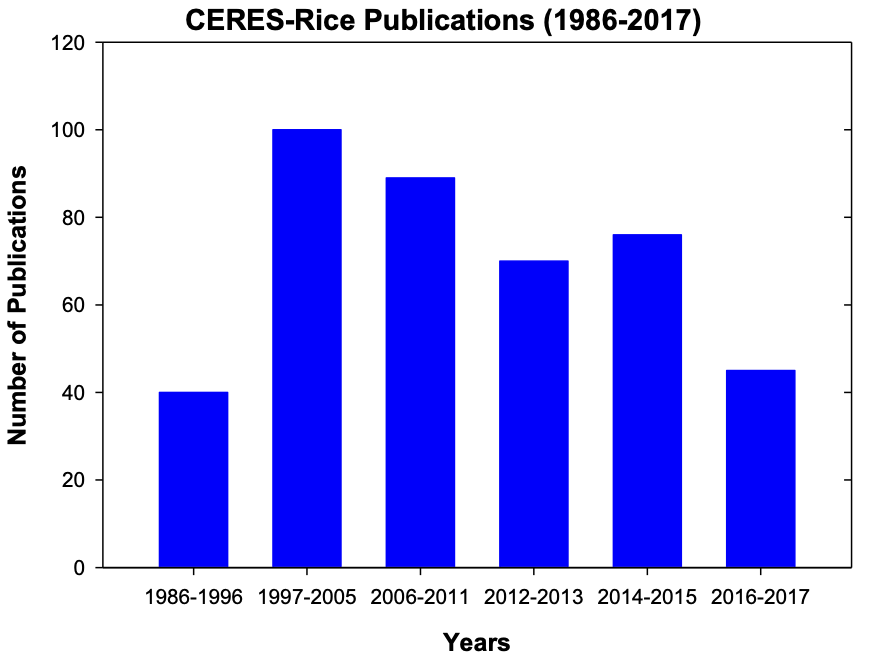
References
- Ahmad, S., Ahmad, A., Soler, C.M.T., Ali, H., Zia-Ul-Haq, M., Anothai, J., Hussain, A., Hoogenboom, G., Hasanuzzaman, M., 2012. Application of the CSM-CERES-Rice model for evaluation of plant density and nitrogen management of fine transplanted rice for an irrigated semiarid environment. Precision Agric 13, 200–218. https://doi.org/10.1007/s11119-011-9238-1
- Ahmad, S., A. Ahmad, H. Ali, A. Hussain, A. Garcia y Garcia, M.A. Khan, M. Zia-Ul-Haq, M. Hasanuzzaman, and G. Hoogenboom. 2013. Application of the CSM-CERES-Rice model for evaluation of plant density and irrigation management of transplanted rice for an irrigated semiarid environment. Irrigation Science 31(3):491-506.
- Ahmad, S., G. Abbas, M. Ahmed, Z. Fatima, M.A. Anjum, G. Rasul, M.A. Khan, and G. Hoogenboom. 2019. Climate warming and management impact on the change of phenology of the rice-wheat cropping system in Punjab, Pakistan. Field Crops Research 230(1):46-61
- Buddhaboon, C., A. Jintrawet, and G. Hoogenboom. 2018. Methodology to estimate rice genetic coefficients for the CSM-CERES-Rice model using GENCALC and GLUE genetic coefficient estimators. The Journal of Agricultural Science 156(4):482-492.
- Buresh, R.J., Singh, U., Godwin, D.C., Ritchie, J.T. and De Datta, S.K., 1991. Simulating soil nitrogen transformations and crop response to nitrogen using the CERES-RICE model. IRRI research paper series-International Rice Research Institute.
- Devkota, K.P, G. Hoogenboom, K. J. Boote, J.P.A. Lamers, M. K. Devkota, and P.L.G. Vlek. 2015. Simulating the impact of water saving irrigation and conservation agriculture practices for rice-wheat systems in the irrigated arid drylands of Central Asia. Agricultural and Forest Meteorology 214–215:266–280.
- Gao, Y., D. Wallach, B.Liu, M. Dingkuhn, K.J. Boote, U. Singh, S. Asseng, T. Kahveci, J. He, R. Zhang, R. Confalonieri, and G. Hoogenboom. 2020. Comparison of three calibration methods for modeling rice phenology. Agricultural and Forest Meteorology 280 (2020) 107785. https://doi.org/10.1016/j.agrformet.2019.107785.
- Godwin, D. C. and Singh, U. 1998. Nitrogen balance and crop response to nitrogen in upland and lowland cropping systems. In: Tsuji, G. Y., Hoogenboom, G. and Thornton, P. K. (Eds), Understanding Options for Agricultural Production. Kluwer Academic Publishers, Dordrecht, the Netherlands, pp. 55–77.
- Godwin, D.C., Meyer, W.S., Singh, U., 1994. Simulation of the effect of chilling injury and nitrogen supply on floret fertility and yield in rice. Aust. J. Exp. Agric. 34, 921–926. https://doi.org/10.1071/ea9940921
- Gupta, R., Mishra, A., 2019. Climate change induced impact and uncertainty of rice yield of agro-ecological zones of India. Agricultural Systems 173, 1–11. https://doi.org/10.1016/j.agsy.2019.01.009
- Hasegawa, T., Li, T., Yin, X., Zhu, Y., Boote, K., Baker, J., Bregaglio, S., Buis, S., Confalonieri, R., Fugice, J., Fumoto, T., Gaydon, D., Kumar, S.N., Lafarge, T., Marcaida Iii, M., Masutomi, Y., Nakagawa, H., Oriol, P., Ruget, F., Singh, U., Tang, L., Tao, F., Wakatsuki, H., Wallach, D., Wang, Y., Wilson, L.T., Yang, L., Yang, Y., Yoshida, H., Zhang, Z., Zhu, J., 2017. Causes of variation among rice models in yield response to CO2 examined with Free-Air CO2 Enrichment and growth chamber experiments. Scientific Reports 7, 1–13. https://doi.org/10.1038/s41598-017-13582-y
- Jha, P.K., P. Athanasiadis, S. Gualdi, A. Trabucco, V. Mereu, V. Shelia, and G. Hoogenboom. 2019. Using daily data from seasonal forecasts in dynamic crop models for crop yield prediction: A case study for rice crop in Nepal’s Terai. Agricultural and Forest Meteorology 265(1):349-358.
- Jones, J.W., G. Hoogenboom, C.H. Porter, K.J. Boote, W.D. Batchelor, L.A. Hunt, P.W. Wilkens, U. Singh, A.J. Gijsman, and J.T. Ritchie. “The DSSAT Cropping System Model.” European Journal of Agronomy 18 (2003): 235–65.
- Kaeomuangmoon, T., A. Jintrawet, C. Chotamonsak, U. Singh, C. Buddhaboon, P. Naoujanon, S. Kongton, Y. Kono, and G. Hoogenboom. 2019. Estimating seasonal fragrant rice production in Thailand using a spatial crop modelling and weather forecasting approach. The Journal of Agricultural Science 157(7). https://doi:10.1017/S0021859619000881
- Li, T., Hasegawa, T., Yin, X., Zhu, Y., Boote, K., Adam, M., Bregaglio, S., Buis, S., Confalonieri, R., Fumoto, T., Gaydon, D., Marcaida, M., Nakagawa, H., Oriol, P., Ruane, A.C., Ruget, F., Singh, B.-, Singh, U., Tang, L., Tao, F., Wilkens, P., Yoshida, H., Zhang, Z., Bouman, B., 2015. Uncertainties in predicting rice yield by current crop models under a wide range of climatic conditions. Global Change Biology 21, 1328–1341. https://doi.org/10.1111/gcb.12758
- Nasir, I.R., F. Rasul, A. Ahmad, H.N. Asghar, and G. Hoogenboom. 2020. Climate change impacts and adaptations for fine, coarse, and hybrid rice using CERES-Rice. Environmental Science and Pollution Research 27:9454–9464.
- Phakamas, N., A. Jintrawet, A. Patanothai, P. Sringam, and G. Hoogenboom. 2013. Estimation of solar radiation based on air temperature and application with the DSSAT v4.5 peanut and rice simulation models in Thailand. Agricultural and Forest Meteorology 180(1):182-193.
- Ritchie, J. T. 1998. Soil water balance and plant water stress. In: Tsuji, G. Y., Hoogenboom, G. and Thornton, P. K. (Eds), Understanding Options for Agricultural Production. Kluwer Academic Publishers, Dordrecht, the Netherlands, pp. 41–54.
- Ritchie, J. T., E. C. Alocilja, U. Singh, and G. Uehara. 1987. IBSNAT and the CERES-RICE model, IN Weatherand Rice, Proceedings of the International Workshop on ‘The Impact of Weather Parameters on Growth and Yield of Rice,” IRRI, Philippines, pp. 271-283.
- Ritchie, J.T. , Singh, U., Godwin, D.C., Bowen, W.T. 1998. Cereal growth, development, and yield. Pages 79-98 in Tsuji G.Y., G. Hoogenboom, and P.K. Thornton (Eds) Understanding options for agricultural production. Kluwer Academic Publishers, Dordrecht, The Netherlands.
- Singh, P.K., Singh, K.K., Rathore, L.S., Baxla, A.K., Bhan, S.C., Gupta, A., Gohain, G.B., Balasubramanian, R., Singh, R.S., Mall, R.K., 2016. Rice (Oryza sativa L.) yield gap using the CERES-rice model of climate variability for different agroclimatic zones of India. Current Science 110, 405–413.
- Singh, U., Ritchie, J.T., 1993. Simulating the Impact of Climate Change on Crop Growth and Nutrient Dynamics using the CERES-Rice Model. Journal of Agricultural Meteorology, 48(5):819-822. https://doi.org/10.2480/agrmet.48.819
- Singh, U., Ritchie, J.T., Godwin, D.C. 1993. A user’s guide to CERES Rice –V2.10.Simulation Manual IFDC-SM-4. IFDC, Muscle Shoals, Alabama, USA.
- Singh, U., Tsuji, G.Y., Godwin, D.C. 1990. Planting new ideas in DSSAT: the CERES-Rice model. Agrotechnology Transfer, 10:1-7. University of Hawaii, Honolulu, Hawaii, USA.
- Vilayvong, S.; Banterng, P.; Patanothai, A.; Pannangpetch, K. Evaluation of CSM-CERES-Rice in simulating the response of lowland rice cultivars to nitrogen application. Aust. J. Crop Sci. 2012, 6, 1534–1541.
- Wallach, D., Nissanka, S.P., Karunaratne, A.S., Weerakoon, W.M.W., Thorburn, P.J., Boote, K.J., Jones, J.W., 2017. Accounting for both parameter and model structure uncertainty in crop model predictions of phenology: A case study on rice. European Journal of Agronomy 88, 53–62. https://doi.org/10.1016/j.eja.2016.05.013
- Zhang, H., Tao, F., Xiao, D., Shi, W., Liu, F., Zhang, S., Liu, Y., Wang, M., Bai, H., 2016. Contributions of climate, varieties, and agronomic management to rice yield change in the past three decades in China. Front. Earth Sci. 10, 315–327. https://doi.org/10.1007/s11707-015-0527-2
- Zhang, J., Miao, Y., Batchelor, W.D., Lu, J., Wang, H., Kang, S., 2018. Improving High-Latitude Rice Nitrogen Management with the CERES-Rice Crop Model. Agronomy 8, 263. https://doi.org/10.3390/agronomy8110263